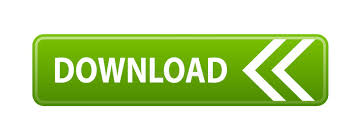
Which states in general that ( X - µ)/ s is normal in the This is a case of the central limit theorem The number of successes is of course discrete, but as n gets large,

> rbinom(1,n,p) # 6 successes in 10 trials To generate binomial numbers, we simply change the value of nįrom 1 to the desired number of trials. There are then two parameters n (the number of Bernoulli trials) and > rbinom(10,n,p) # 10 different such numbersĪ binomially distributed number is the same as the number of 1's in
#Pick a number between 1 and 3 10 times trial
Success or failure, success with probability p.Ī single Bernoulli trial is given with n=1 in the binomial N independent Bernoulli trials where a Bernoulli trial results in They have the distribution of the number of successes in The binomial random numbers are discrete random # also for IQs using rnorm(100,mean=100,sd=16)īinomial. > hist(x,probability=TRUE,col=gray(.9),main="normal mu=0,sigma=1") To see the shape for the defaults (mean 0, standard deviation 1) we One specifies the mean and the standard deviation. Here the function is called as rnorm(n,mean=0,sd=1) where 270.4325 # how long for a baby (10 days early) To normal with mean 0 (centered) and variance 1. The family of normals can be standardized Standard deviation about 10 (approximately). IQs may be normally distributed with mean 100 and standard deviationġ6, Human gestation may be normal with mean 280 and These are the location and spread parameters. Normal numbers are the backbone of classical statistical theoryĭue to the central limit theorem The normalĭistribution has two parameters a mean µ and a standard deviation Notice how the dunif function was used with the The only tricky thing was plotting the histogram with a background > hist(x,probability=TRUE,col=gray(.9),main="uniform on ") To see the distribution with min=0 and max=1 (the The general form is runif(n,min=0,max=1) which allows you toĭecide how many uniform random numbers you want ( n), and the For example P( a runif(1,0,2) # time at light Some function which specifies the probability that a random number Numbers ranging from familiar families of distributions to specializedĦ.1 Random number generators in R- the ``r'' functions.Īs we know, random numbers are described by a distribution. In fact, R can create lots of different types of random

> RollDie = function(n) sample(1:6,n,replace=T) Files contents_motif.gif DESCRIPTION index.html next_motif.gif PACKAGES previous_motif.gif R-logo.gif Simple_0.6.tar.gz Simple_0.6.zip simpleR.R stat001.gif stat001.html stat002.gif stat002.html stat003.gif stat003.html stat004.gif stat004.html stat005.gif stat005.html stat006.gif stat006.html stat007.gif stat007.html stat008.gif stat008.html stat009.gif stat009.html stat010.gif stat010.html stat011.gif stat011.html stat012.gif stat012.html stat013.gif stat013.html stat014.gif stat014.html stat015.gif stat015.html stat016.gif stat016.html stat017.gif stat017.html stat018.gif stat018.html stat019.gif stat019.html stat020.gif stat020.html stat021.gif stat021.html stat022.gif stat022.html stat023.gif stat023.html stat024.gif stat024.html stat025.gif stat025.html stat026.gif stat026.html stat027.gif stat028.gif stat029.gif stat030.gif stat031.gif stat032.gif stat033.gif stat034.gif stat035.gif stat036.gif stat037.gif stat038.gif stat039.gif stat040.gif stat041.gif stat042.gif stat043.gif stat044.gif stat045.gif stat046.gif stat047.gif stat048.gif stat049.gif stat050.gif stat051.gif stat052.gif stat053.gif stat054.gif stat055.gif stat056.gif stat057.gif stat058.gif stat059.gif stat.htmlĪlthough Einstein said that god does not play dice, R can.
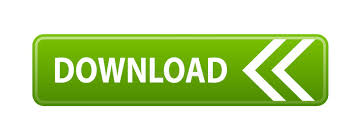